9 billion regressions: A multiverse approach to statistical analysis
Model uncertainty is a central challenge of modern science. Researchers do not know what model actually generated their data and they cannot be sure if the statistical method they use is best. Moreover, researchers can explore innumerable analytic variations in their search for results, but readers can only see the handful of estimates curated for publication. These researcher ‘degrees of freedom’ represent the crisis in science today: are empirical conclusions driven mostly by the data, or mostly by the analytic assumptions made by researchers? How much influence does a researcher actually have over the results?
Dr Cristobal Young, Associate Professor of Sociology at Cornell University, is addressing this conundrum. He believes that “the ‘crisis in science’ today is rooted in problems of model uncertainty and weak transparency.” Dr Young demonstrates the leeway currently afforded to researchers and authors, enabling them to choose their preferred results. He also offers methods to quantify the influence of the researcher’s choice of statistical model.
Model uncertainty
All empirical estimates require specific modelling assumptions, and the researcher has to choose which assumptions to employ. Decisions such as defining variables, cleaning data, the treatment of outliers, the calculation of standard errors, and the choice of functional form mean that competent researchers can choose different statistical methods and produce different results from the same dataset. Designing the analysis and specifying the model often pose the biggest challenges to researchers. Dr Young points out that the underlying issue here is model uncertainty, in that no one actually knows which model specification is best for a particular study. There are often many credible models that might offer a variety of results that the data could support. This makes it difficult to say whether a particular conclusion would be reached by all reasonable researchers analysing a particular dataset, or if the empirical results are highly dependent on the researcher’s particular choices. This underlines the importance of transparency in model specification.
Multimodel analysis
Rather than asking ‘which is the best model?’ Dr Young encourages researchers to ask ‘which models deserve consideration?’ Embracing a wider range of models allows authors, journal reviewers and readers a clearer view into how the conclusions rest on a credible, comprehensive, and robust foundation. Multimodel analysis acknowledges alternative combinations of modelling assumptions that different authors could use and offers significant improvements in the transparency and robustness of statistical research. The fundamental challenge in multimodel analysis, however, is defining the set of plausible models.
The ‘crisis in science’ today is rooted in problems of model uncertainty and limited transparency.
The multiverse of model assumptions
Dr Young has developed a computational framework for multimodel analysis. He explains that the goal is “to offer transparent and systematic reporting of alternative results that a researcher could obtain from other plausible model specifications.” In a sense, there is a multiverse – multiple universes of analysis – in which all the credible ways of analysing a research question with a given data set all exist at once. A computational approach aims to reduce the author’s discretion in choosing their preferred result from the multiverse, while also expanding the range of models and results under consideration.

The method involves specifying a set of plausible model components, such as control variables, variable definitions, estimation commands, functional forms, and standard error calculations, and estimating all possible combinations of these components. This can involve running thousands of small variations of model specifications with the results reported as a graphical distribution of estimates.
In empirical testing, the multiverse shows three basic patterns of model robustness:
• In some cases, a statistical result holds regardless of how the model is specified. Any combination of the model components will generate the same result.
• Other times, a result depends on only one or two model components, for instance a particular control variable. This prompts follow-up analyses to find out why the control variable is so important.
• Most worrisome, some results depend on a ‘knife edge’ specification, and are supported in only one in a 100 plausible models.
Authors are still free to present their preferred model, albeit within the context of a graph displaying other results that can be found with the set of acceptable model components.
9 billion regressions
False positives – statistically significant results – are common even when analysing data that are made entirely of random noise. Dr Young’s research employs large-scale random noise simulations to investigate the issue of excess false positive errors occurring from model uncertainty. Moreover, it shows how a multiverse analysis can identify and eliminate many false positives.
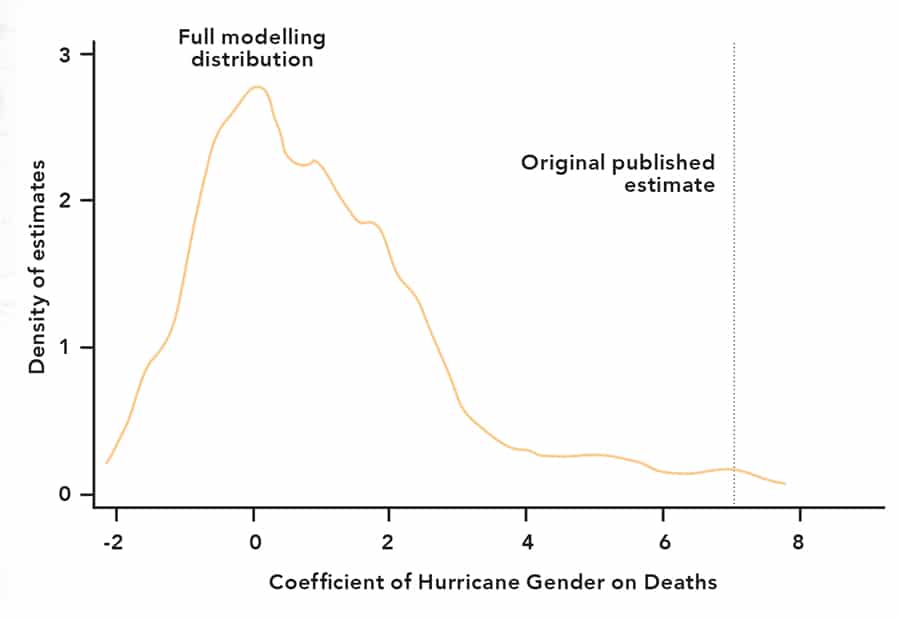
A multiverse analysis using 1,152 alternative models largely finds no effect.
The simulation strategy involved conducting noise-on-noise regression analysis. This work involved completing 5,000 iterations of the simulation for each of the 17 unique conditions for data size and degree of model uncertainty. Approximately 550 million regressions were carried out for each condition – more than 9 billion in total. The simulations show that the proportion of false positives is highest when the sample size is low and model uncertainty is high. When the false positive rate is highest, multiverse analysis works best – routinely flagging ‘significant’ results as being non-robust and continent on using an extremely idiosyncratic model specification.
When evaluating research findings, a multiverse analysis of alternative models is at least as important
as statistical significance.
These simulations indicate that, first, to mitigate the proliferation of false positive results, researchers should aspire to use larger data sets. Second, mature research areas with strong, well-established prior theory – and less model uncertainty – will tend to produce fewer false positives. Finally, multiverse analysis provides a strong check against inflated rates of statistical significance in more novel research areas where there is greater uncertainty in the analysis.
Multiverse in the real world
The informative potential of multiverse analysis is illustrated by revisiting a controversial study reporting that hurricanes with female names are more deadly. Using data on all hurricanes making landfall in the U.S. over six decades, the study reports that hurricanes with feminine-sounding names consistently had higher death tolls. The study inspired a long series of comments and rejoinders published in the original journal, and many alternative estimation strategies were discussed. Dr Young used all possible combinations of those suggestions to populate a multiverse analysis comprising 1,152 unique model specifications. The result was telling: the vast majority of plausible models show a zero effect of hurricane ‘gender,’ and less than 5 percent of specifications supported the original claims. Almost any other way of analysing the data failed to support the study’s claims.
Dr Young recommends carrying out model robustness analysis on empirical data to help distinguish between true relationships and suspect findings in order to have more empirically defensible conclusions – findings based on the data rather than the modelling assumptions.
The next frontier: some models are more equal than others
The multiverse methodology considers all possible combinations of plausible models and produces an associated distribution of estimates. Each model is treated as equally valid when computing multiverse statistics and distributions. Dr Young notes, however, that some of the plausible models are more compelling than others. This raises the possibility of weighting models by appropriate measures of validity. Young’s work on this has explored the use of “model influence” scores to weight possible models, taking into account potential omitted variable bias. He cautions that the weighting of possible models involves a trade-off between transparency and model selection. However, it offers a promising path forward for multiverse analysis.
Dr Young’s computational framework makes the multiverse central to how analyses are conducted and reported. It facilitates more rigorous and comprehensive robustness testing and enables model uncertainty to be embraced as an inherent part of social science. It reduces the fundamental asymmetry of information between analyst and reader, and addresses the overabundance of false positive research findings. Dr Young stresses that “when evaluating research findings, a multiverse analysis of alternative models is at least as important as statistical significance.”
Note: Dr Young’s multiverse analysis software is open source and freely available to researchers. Type ssc describe mrobust in Stata.
Personal Response
What are your plans for future research involving multiverse analysis?
I am planning to write a book, tentatively titled “Into the Multiverse: Computational Methods for Robust Research.”