Is it possible to train medical experts using Artificial Intelligence (AI) training methods?
Previous research has shown that human beings can be trained to recognise visual patterns in images. But can non-professionals be trained to recognise subtle, abstract patterns in images that usually takes a professional expert to recognise? This is an area of science, called expertise development, that fascinates Dr Jay Hegdé from the Department of Neuroscience and Regenerative Medicine at Augusta University. He has set out to address this poorly understood topic by exploring the world of camouflage breaking and medical image perception.
Finding hidden objects in a visual scene
The ability to find hidden targets in complex backgrounds is critically important in activities such as hunting and warfare – indeed, it can often be a matter of life and death in both cases. Camouflage represents an extreme case of this, whereby a target object is effectively disguised against its background, making it hard to distinguish even when it is hiding in plain sight. From a computational viewpoint, camouflage breaking is one of the most difficult object recognition tasks.
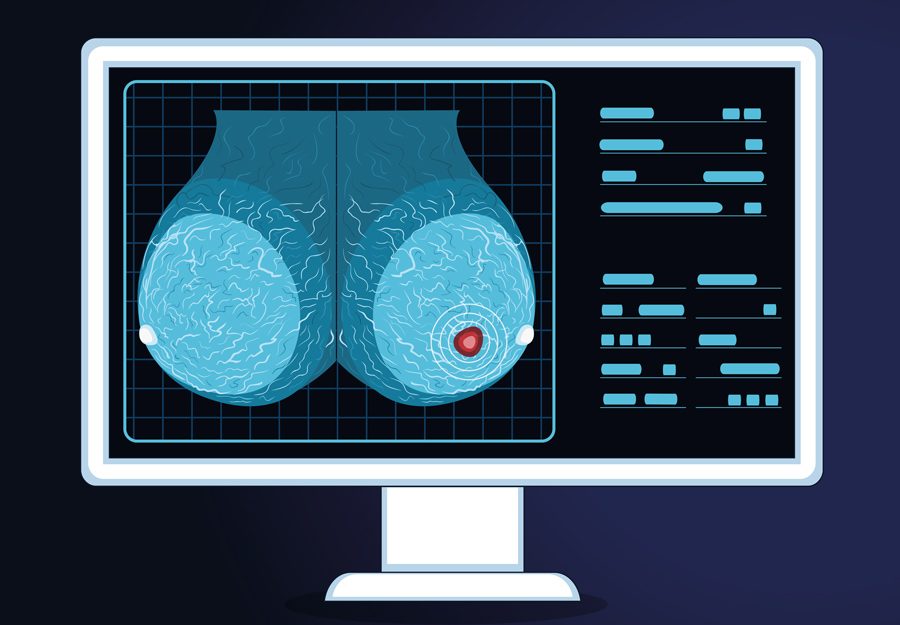
In Artificial Intelligence (AI), smart computers learn to perform everyday tasks from a large number of examples relevant to the task. For instance, you can train your smartphone to recognise your voice by repeating a sentence many times. From these examples, your smartphone learns what you sound like. Similarly, self-driving cars are taught to recognise road signs and traffic conditions by providing them with a large number of relevant examples. This learning strategy is called deep learning (DL).
In his earlier work, Dr Hegdé and his team sought to use DL to train ordinary human subjects to perform complex visual tasks that are usually performed by highly trained experts. To prove the principle that naive subjects can be trained using DL methodology to begin with, they used DL to train ordinary people to break camouflage by showing them a large number of digitally created camouflaged scenes. Indeed, at the end of training, the previously novice subjects were able to break camouflage with a very high degree of accuracy.
Highly skilled doctors can look at medical images and immediately recognise subtle visual patterns that flag advanced warning of disease.
Additional experiments by his team revealed a somewhat counterintuitive principle as to how the brain learns to break camouflage – i.e., recognising a foreground object camouflaged against its background. It does so by learning the background. That is, the brain learns the statistical properties of the background from a large number of examples, so that it knows what the background ‘looks like’. When the image has a foreground object hidden in the background, the image will have slightly different statistics, by virtue of the added foreground object. Therefore, the brain can tell that there is a proverbial ‘odd man out’ – an object that does not belong with the background.
To reflect the similarity of this approach with the DL methods used in AI, Dr Hegdé coined the term ‘human deep learning’. Encouraged by the success of his camouflage training study, he wanted to extend it to another area where visual pattern recognition is vital: examining a mammogram to detect breast cancer in medical images.

Intuitively spotting breast cancer
In the fields of radiology and pathology, highly skilled doctors can look at medical images and immediately recognise subtle visual patterns that flag advanced warning of disease such as cancer – often before any detectable physical manifestation occurs. How does the brain of a trained radiologist, for example, learn to detect these patterns? Is it possible to train novices to similarly detect subtle visual patterns by utilising ‘human deep learning’? Dr Hegdé hoped that his previous findings in camouflage breaking might help answer these questions. They formed the basis of his next investigation into mammography. For this study, Dr Hegdé and his team used those mammograms for which the ‘ground truth’ had been established; that is, whether or not the patient actually had breast cancer had been independently established.
Selecting the mammograms for the study
The investigators used two categories of mammograms from a public database called the Digital Database for Screening Mammography (DDSM). The first category of 632 ‘cancer’ mammograms all had signs of cancer in them. No images that didn’t show or hinted at the possibility of cancer were used. In each case, the region of interest (ROI) containing the cancer had been previously demarcated by expert radiologists. In these mammograms, the ROI in question had either tiny calcium deposits called microcalcification (63% of the cases) or breast mass (37% of the cases), but not both.
The team also selected a different set of 632 unique, ‘non-cancer’ mammograms categorised as ‘benign’. In these cases, the mammogram was ambiguous enough to necessitate patient call-back, but ultimately no cancer was found.
Testing, training, and testing again
Dr Hegdé and his team used a similar set of DL training and testing procedures as they had used in the earlier camouflage study. The method involved three successive phases: a pre-training test, a training phase and, finally, a post-training test. In these medical experiments, normal-sighted, adult volunteers (or ‘subjects’) with no previous medical or imaging background were used. They ranged in ages between 18-65.
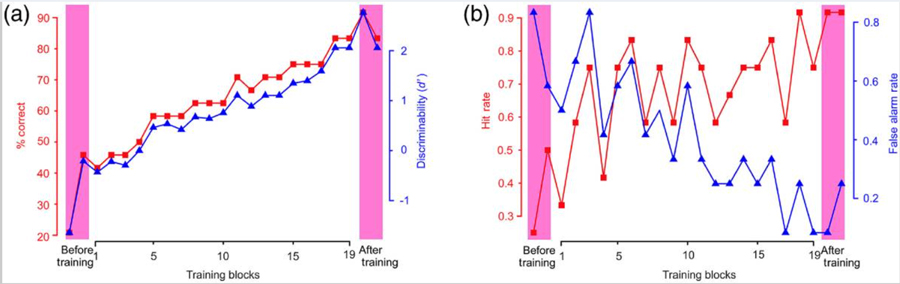
Each of the three phases of the study consisted of a series of trials one after the other. During each trial, the subject was shown one mammogram selected at random. The single, explicit task for the volunteer was to simply report the presence or absence of a cancer in any given image by pressing a designated key on the computer keyboard.
Quantifying eye positions during visual scrutiny
At the start of each trial, the subject had to gaze briefly at the same central point on the computer screen, something that could be checked by high-resolution eye tracking. After this, the subjects were allowed to freely move their eyes, but the position of their eyes were tracked throughout the trial. This was undertaken because when a viewer actively scrutinises an image that is of interest to them, their eye frequently changes the point of gaze. During reading, for instance, there are small movements, but when we survey a room these movements are greatly amplified. These ballistic-type movements are called ‘saccades’. When we gaze steadily at portion of the image to scrutinise it closely, the resulting tiny eye movements are termed ‘microsaccades’. The team wanted to monitor any eye movement changes during the course of training as they would help characterise any training-dependent changes in the subject’s ability to read the images.
Learning in their own fashion
Subjects were randomly shown mammograms and asked whether cancer was evident in the image or not. For the pre-training test, 24 ‘with cancer’ mammograms were randomly selected and another 24 without. For the training phase that followed, none of the previous samples were used. This helped to remove any ‘above chance’ level results due to having memory of an image that the subject had previously seen. What’s important to understand is that the subjects were not told what to learn or how to learn during the training phase. The only information they received was the image (that is, the mammogram) itself and feedback if they gave the correct diagnosis. This was important because the training methodology needed to meet the functional criteria of DL.
The team conducted some other experiments to further improve the training outcomes. One involved allowing the subjects to view a mammogram again (‘review phase’) after they made their initial report and received the feedback, but with ROI digitally superimposed on the mammogram. During the review phase in another, similar experiment, radiologically vetted diagnosis and diagnostic information were also presented (along with the ROI outlined mammogram), allowing re-examination in light of this additional information as well.
Without training, understandably enough, the subjects reported mammograms with prominent parts, such as breast densities, as cancerous even though they weren’t. Learning the difference takes time. Although a thorough understanding of what exactly is learned in DL and how it is learned remains elusive, monitoring eye movement may hold the key to unlocking such understanding. What is certain is that the training-dependent improvement in performance was significant across all volunteers as a group and for each individual volunteer in the study.
The same deep learning methods driving smart computers can be used to train novices to detect patterns of breast cancer in mammograms.
The patterns of microsaccades also changed as the subjects got better at detecting cancer. In particular, the number of microsaccades decreased as the subjects got better. At the start of the training, microsaccades were directed at (that is, the subjects gazed at) visually prominent portions of the mammogram. As training progressed, subjects tended to gaze at subtler and less visually prominent regions that were more diagnostic of cancer, and reached decisions quicker. After the training, gazes landed much more frequently within the ROI.
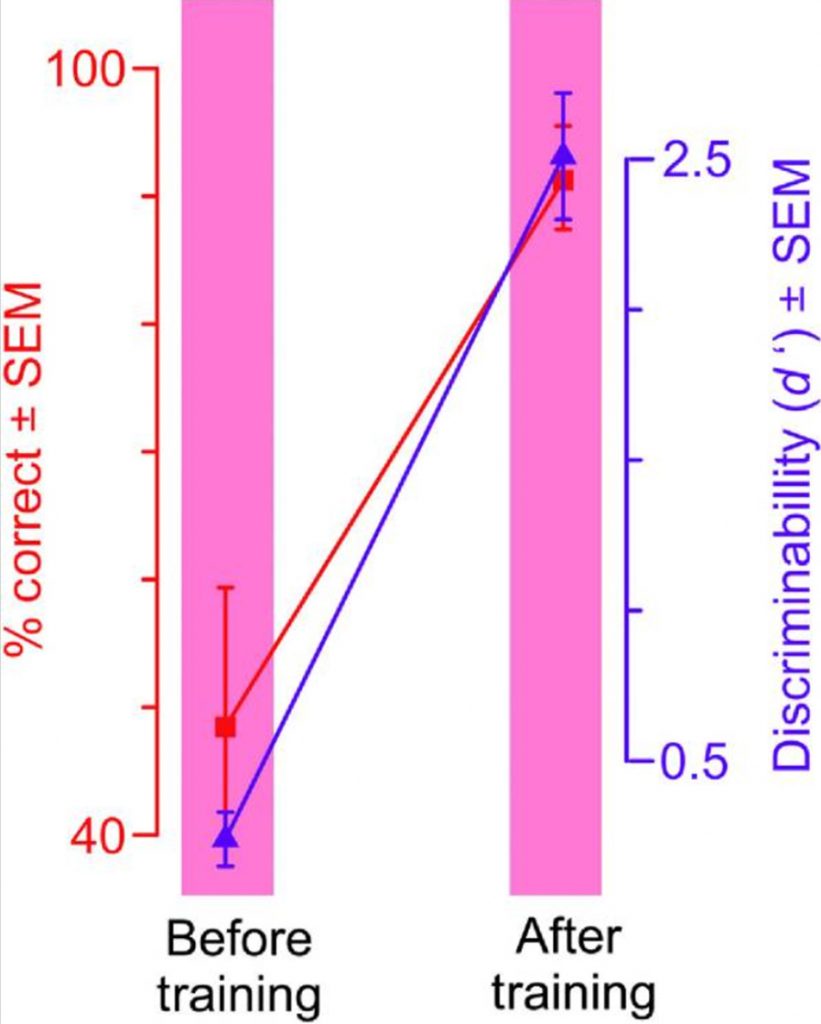
Photo Credit: https://pubmed.ncbi.nlm.nih.gov/32042860/
The researchers found that the more information the subjects were given, the better their cancer detection performance became. Collectively, the results provide substantial evidence that, in principle, the same DL methods driving smart computers can also be used to train novices to detect diagnostic image patterns of breast cancer in mammograms.
Human expertise learning
The principle that complex visual patterns can be deep-learned from examples is not new. Still, by leveraging lines of work such as camouflage breaking and mammography as exemplar cases, Dr Hegdé and his team have demonstrated that DL techniques can, in principle, be used to train and have practical potential in human expertise learning.
In recognising diagnostic visual patterns of microcalcifications and breast masses in mammograms, they highlight the fact that expertise in visual pattern recognition can be acquired without any medical expertise or even any particular a priori aptitude for complex pattern recognition, raising important implications for medical education in the process.
Dr Hegdé cautions that these results by no means imply that the subjects in this study are as good as practicing radiologists. He emphasises that there is whole lot more to being a radiologist than just learning visual patterns. Besides, the visual patterns of breast cancer that the subjects had to learn, microcalcifications and breast masses, while quite difficult to learn, are still some of the easiest breast cancer patterns.
Personal Response
Where do you intend to go to next? What refinements could you make to enhance the learning?
We are currently trying to answer three questions raised by this study. First, what are the changes that occur in the brain as subjects learn to do this task? We are using brain imaging to answer this question. Second, what exactly about the mammograms do the subjects learn when they learn to do this task, and is it the same as what radiologists themselves learn and use? We are using methods from mathematical psychology to answer this question. Finally, we have constructed neural networks in a computer that do similar tasks. We are systematically comparing the behaviour of this network with that of the subjects before, during and after the training.